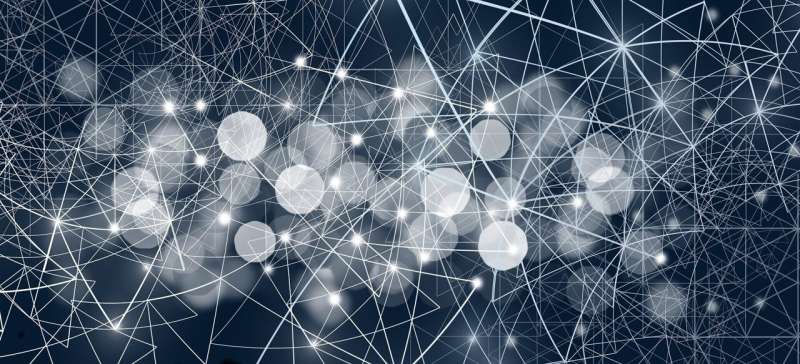
Imagine developing a finer control knob for artificial intelligence (AI) applications like Google Gemini and OpenAI ChatGPT.
Mikhail Belkin, a professor with UC San Diego’s Halıcıoğlu Data Science Institute (HDSI)—part of the School of Computing, Information and Data Sciences (SCIDS)—has been working with a team that has done just that. Specifically, the researchers have discovered a method that allows for more precise steering and modification of large language models (LLMs)—the powerful AI systems behind tools like Gemini and ChatGPT. Belkin said that this breakthrough could lead to safer, more reliable and more adaptable AI.
The research relies on recent work that has been published in Science and Proceedings of the National Academy of Sciences.
“Currently, while LLMs demonstrate impressive abilities in generating text, translating languages and answering questions, their behavior can sometimes be unpredictable or even harmful,” Belkin said. “They might produce biased content, spread misinformation or exhibit toxic language.”
The multi-institutional research team includes Belkin, Daniel Beaglehole (Computer Science and Engineering Department at UC San Diego Jacobs School of Engineering), Adityanarayanan Radhakrishnan (Broad Institute of MIT and Harvard SEAS) and Enric Boix-Adserà (MIT Mathematics and Harvard CMSA).
Belkin said that they tackled this challenge by developing a novel “nonlinear feature learning” method. This technique allowed them to identify and manipulate important underlying features within the LLM’s complex network.
Think of it as understanding the individual ingredients in a cake rather than just the final product. By understanding these core components, the researchers then guided the AI app’s output in more desirable directions.
“It’s like we’re gaining a deeper understanding of the AI app’s internal thought process,” Belkin explained. “This allows us to not only predict what kind of outputs the model will generate, but also to actively influence it towards more helpful and less harmful responses.”
Their approach involved analyzing the internal activations of the LLM across different layers. This allowed them to pinpoint which features are responsible for specific concepts, such as toxicity or factual accuracy. Once these features were identified, the researchers adjusted them to encourage or discourage certain behaviors.
The team demonstrated the effectiveness of their method across a range of tasks, including detecting and mitigating hallucinations (instances where the AI generates false information), harmfulness and toxicity. They also showed that their technique could steer LLMs to better understand concepts in various languages, including Shakespearean English and poetic language.
“One of the significant benefits of this new method is its potential to make LLMs more efficient and cost-effective,” Belkin said. “By focusing on the crucial internal features, we believe that we can fine-tune these powerful models using less data and computational resources—this could, in turn, make advanced AI technology more accessible.”
This type of research also has the potential of opening doors for creating more tailored AI applications. Imagine an AI assistant specifically designed to provide accurate medical information or a creative writing tool that avoids clichés and harmful stereotypes. The ability to precisely steer LLMs brings these possibilities closer to reality.
The researchers have made their code publicly available—encouraging further exploration and development in this critical area of AI safety and control.
“As LLMs become increasingly integrated into our daily lives, being able to understand and guide their behavior is paramount,” said Rajesh Gupta, who is the interim dean for SCIDS, the HDSI founding director and a distinguished professor with the Computer Science and Engineering Department at UC San Diego Jacobs School of Engineering.
“This new research by Professor Belkin and team represents a significant step towards building more reliable, trustworthy and beneficial artificial intelligence for everyone.”
More information:
Adityanarayanan Radhakrishnan et al, Linear Recursive Feature Machines provably recover low-rank matrices, Proceedings of the National Academy of Sciences (2025). DOI: 10.1073/pnas.2411325122
Adityanarayanan Radhakrishnan et al, Mechanism for feature learning in neural networks and backpropagation-free machine learning models, Science (2024). DOI: 10.1126/science.adi5639
Citation:
Steering AI: New technique offers more control over large language models (2025, May 14)
retrieved 14 May 2025
from
This document is subject to copyright. Apart from any fair dealing for the purpose of private study or research, no
part may be reproduced without the written permission. The content is provided for information purposes only.
Leave a comment